What is a Data Lake?
A data lake is a system or repository of data stored in its natural/ raw format, usually, object blobs or files. A data lake is usually a single store of all enterprise data including raw copies of source system data and transformed data used for tasks such as reporting, visualization, advanced analytics, and machine learning. (source Wikipedia)
It is no longer a secret that the digital universe that meets the data needs of most businesses is growing exponentially. Most companies generate too little or no added value from this data.
In this environment, it is critical that companies use data analytics to increase their competitiveness and fulfill the requirements of “information generation.” For example, storing and analyzing company-wide data enables you to predict your purchasing behavior, improve customer service, or increase productivity in your various business units. Big data analysis should no longer be a requirement but a necessity. Already today, there are tools for parallel processing of large amounts of data and AI-based technologies, such as, e.g., Machine Learning, Deep Learning, NLP – Natural Language Processing, etc., which enable the highly efficient analysis of company data.
The Evolution of Analytics
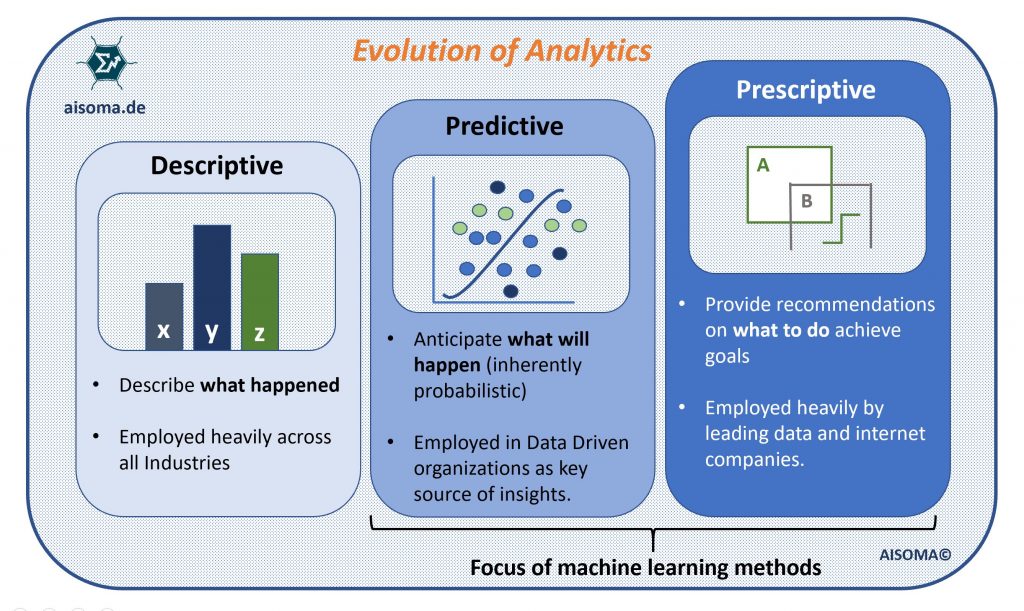
Many organizations, however, get stuck early on the journey. One of the main reasons for this is that IT and the rest of the business are not always focused on the best use cases and business goals of big data projects. While some companies are experimenting with fundamental data analysis (and some have not even begun to do so), many are just not prepared to the next level, which is much more complex and detailed.
[bctt tweet=”4 Signs your Company should Invest in a Data Lake? #DataLake #BigData #MachineLearning #AI #DataDriven #DataDriven #DataStrategy” username=”AISOMA_AG”]
How does a company know when to invest in a Data Lake?
There are four tell-tale signs:
Operational complexity
If a company attempts to scale its infrastructure but has no option for additional full-employee equivalent (FTE) support, then in a Pre Data Lake environment there is a high probability that the data requirements will exceed the company’s ability to manage them. Traditional 1-tier data resources are not always pooled virtually, which limits the amount of memory that a single administrator can handle and thus provides a clear argument for a more flexible shared memory resource.
Operating cost
If a business finds that IT business needs continue to increase as it seeks to lower its cost of ownership, then it’s time to look at a new approach. The same operational overheads that limit the ability for additional FTEs also lead to growing IT operational resource management costs. Companies either need more RTDs or need to invest additional support in third-party support to monitor, manage, deploy, and improve their systems. The latter approach scales an order of magnitude better – or more – than merely increasing headcount.
Production strain
Another important indicator of the need for a data lake is when existing analytics applications burden a company’s production systems. Real-time analytics can be extremely resource-intensive, regardless of whether you want to gain insights through video analytics, HD video streams, or surf through a massive flow of content. Dedicated resources are needed to keep users from slowing down when using production systems. Data holes are the key to ensuring that real-time analytics can run at optimal performance.
Multi-protocol analysis
An important definitive indicator that a business needs a data lake is when data scientists run apps on a variety of Hadoop distributions and need to attach their data to them. Companies will need multi-protocol support in the future if data analytics is to be expanded, and they must plan this with a Datapoint strategy.
Departments such as marketing have taken a leading role in the introduction of big data analytics. To better understand their customers, they have used the insights gained from the analyzes and optimized their communication accordingly. But other business areas from HR to IT to business planning and beyond are now interested in the benefits of big data analytics.
From the finance industry to retail, manufacturing, and media companies, it is believed across the industry that their problems, challenges, and opportunities are unique. But when you abstract the details, you’re always coming back to the same universal challenges mentioned in this article. All this unites and characterizes the transformation of information technology and the potential of big data analytics.
Not every company will be prepared to carry out analyses of large amounts of data. But most will have to start with planning at least. Otherwise, you risk losing customers to the competition because they are already using the new analysis options.
Businesses should embrace the opportunities of big data analytics, and machine learning, in particular, to add value from their data. Those companies that do not even plan to do so will no longer be able to maintain their market position in the age of digitization.
Future of Data Science
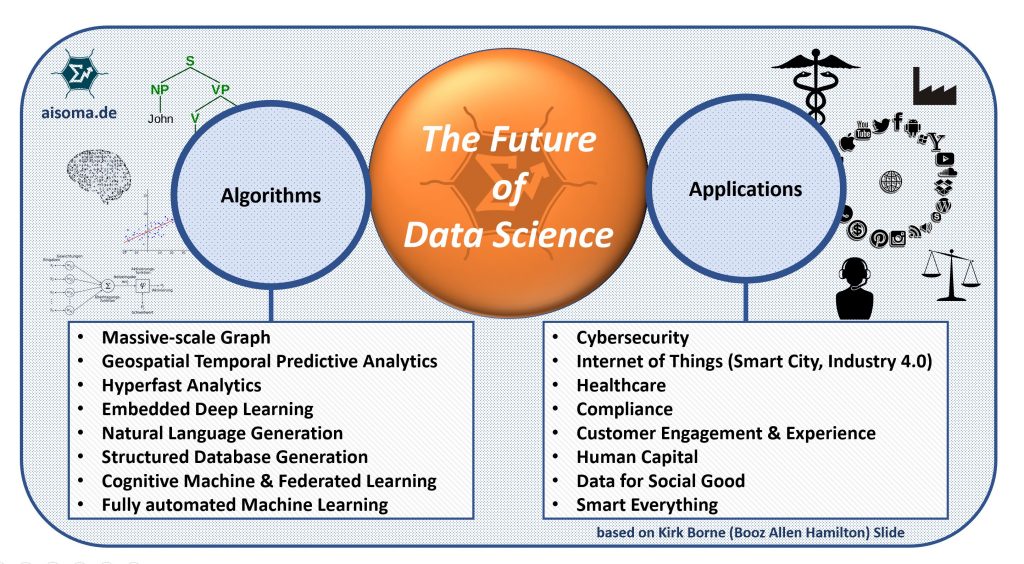
The establishment of a data lake is an essential prerequisite for the implementation of successful AI projects.
Read also: 10 Predictive Analytics Use Cases
AISOMA