Industrial Intelligence was one of the core topics at this year’s Hannover Fair. Due to the importance of this area, we took a closer look at the topic area.
The definition of Industrial Intelligence according to the Hannover Messe is as follows:
Industry 4.0 meets AI
Artificial intelligence has the potential to revolutionize the production and energy industries. People teach machines to act logically and purposefully to meet customer needs. AI systems generate knowledge and today, based on data and algorithms, can continuously optimize operating states or reliably predict faults and failures – in production processes, in the power grid or in logistics. That’s what Integrated Industry – Industrial Intelligence stands for. (Source)
Industrial Intelligence can be divided into 3 categories.
3 Categories of Industrial Intelligence
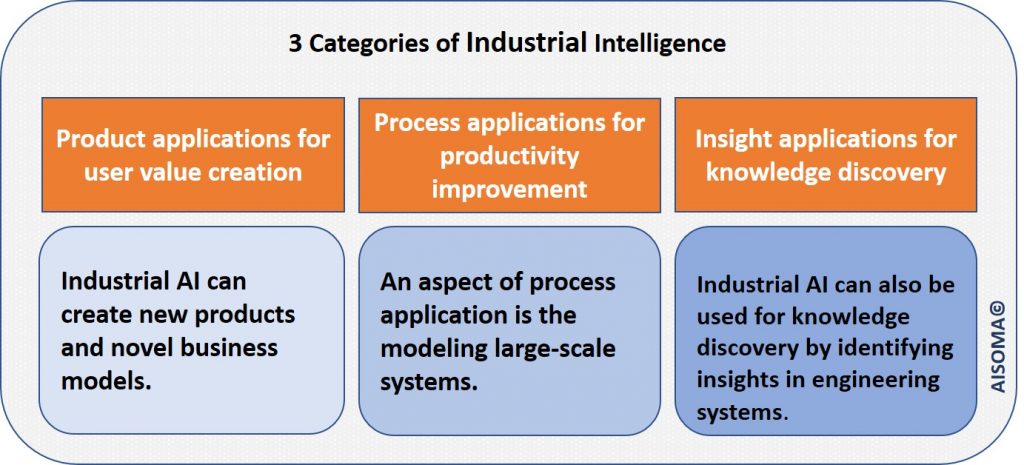
1. Product applications for user value creation
Industrial AI can be embedded in existing products or services to make them more effective, reliable, safer, and to enhance their longevity. The automotive industry, for example, uses computer vision to avoid accidents and enable vehicles to stay in lane, facilitating safer driving. In manufacturing, one example is the prediction of blade life for self-aware band saw machines, so that users will be able to rely on evidence of degradation rather than experience, which is safer, will extend blade life, and build up blade usage profile to help blade selection.
Industrial AI can create new products and novel business models.
2. Process applications for productivity improvement
Automation is one of the major aspects of process applications of industrial AI. With the help of AI, the scope and pace of automation have been fundamentally changed. AI technologies boost performance and expand the capability of conventional AI applications. An example is collaborative robots. Collaborative robotic arms are able to learn the motion and path demonstrated by human operators and perform the same task. AI also automates the process that used to require human participation. An example is the Hong Kong subway, where an AI program decides the distribution and job scheduling of engineers with more efficiency and reliability than human counterparts do.
Another aspect of process application is the modeling of large-scale systems.
3. Insight applications for knowledge discovery
Industrial AI can also be used for knowledge discovery by identifying insights in engineering systems. In aviation and aeronautics, AI has been playing a vital role in many critical areas, one of which is safety assurance and root cause. NASA is trying to proactively manage risks to aircraft safety by analyzing flight numeric data and text reports in parallel to not only detect anomalies but also relate it to the causal factors. This mined insight into why certain faults happen in the past will shed light on predictions of similar incidents in the future and prevent problems before they occur.
Predictive and preventive maintenance through data-driven machine learning is also critical in cost reduction for industrial applications. Prognostics and health management (PHM) programs capture the opportunities at the shop floor by modeling equipment health degradation. The obtained information can be used for efficiency improvement and quality improvement
The data-based integration of all value-added processes is the decisive factor for the competitiveness of the manufacturing industry.
[bctt tweet=”3 Categories of Industrial Intelligence #IndustrialIntelligence #IoT #industry40 #PredictiveMaintenance #EdgeComputing #Manufacturing #Analytics #AI #ML” username=”AISOMA_AG”]
If you have any questions, please do not hesitate to contact us.
AISOMA
Recommended article: 5 Variations of AI