In many areas of business (e.g. finance, marketing), machine leaning is becoming a popular method for data analysis. So, what is machine learning and what can machine learning offer the chemical industry?
What is machine learning?
Essentially, machine learning is a method of creating a model based on sample data and then using the model to make predictions or to recognise patterns in data. Or to put it another way, it is the act of teaching computers to make and improve predictions based on data.
Two popular examples of machine learning are support vector machine (SVM) and artificial neural network (ANN) systems (Figure 1). SVM is used in regression and classification problems to place data into groups with distinct features, and ANN uses a large number of interconnected neurons that use numerically weighting to specify how different neurons are connected.
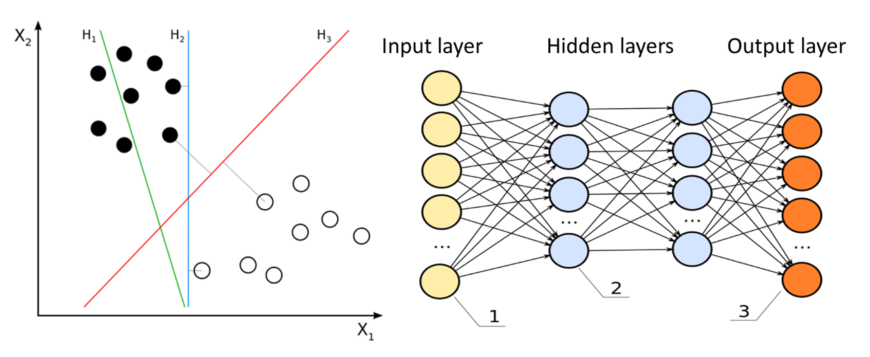
Figure: On the left is an example of a SVM were the red line has divided the data into two groups (image by ZackWeinberg), and on the right, is an example of an ANN used to perform (image by Zufzzi).
Use cases in the chemical industry
Machine learning for designing new materials
Traditional methods of developing new materials are highly time and resource intensive [1]. The discovery and design of new materials with novel properties aided by machine learning techniques is becoming a hot topic. For example, ANN modelling has found a place in applications such as the prediction of material melting points [2], and the density and viscosity of biofuel compounds [3].
Machine learning techniques are also being used to simulate the strength of concrete materials a useful application for civil construction projects [4], to design lithium-ion batteries useful for improving energy efficiency [5], and to identify relationships between temperature, composition, and mechanical properties in polymer-clay nanocomposites [6]. In the realm of materials science, an investment into machine learning has the potential to speed up development processes and to improve predictive models.
Machine learning in quantum chemistry
In the search for novel materials, quantum chemical modelling and simulation is taking an increasingly important role [7]. The major issue is the computational effort required that increases with system size, meaning that to generate a numerical solution that is in agreement with an experimental one is limited to small systems. The use of machine learning techniques to supplement traditional quantum calculations is gaining interest from quantum chemists for problems in materials science, organic chemistry, and biochemistry. Here, machine learning can be used to interpolate between reference calculations in the hope of leading to substantial computational savings [8].
Recent works include a machine learning algorithm based on non-linear statistical regression to predict the atomization energies of organic molecules [9], and a multi-task deep neural network model that predicted atomization energies and several other electronic ground and excited state properties [10]. In the future, models that combine quantum mechanics with machine learning have the potential to deliver the accuracy of the former with the speed of the latter.
Machine learning in predicting biological activity
Predicting the biological activity of chemicals has a long history in both biomedical and environmental research in terms of drug design and predicting toxicity. Bioactivity profiling using high-throughput in vitro assays can reduce the cost and time required for toxicological screening of chemicals and can also reduce the need for animal testing. Other approaches include predicting the toxicity of chemicals using structure−activity relationship modelling, a technique which is used to predict biological activity based on the chemical structure of a compound.
Examples of currently used models include the BIOWIN module contained with EPISuite [11], a fragment-based model, that evaluates a chemicals molecular features to estimate aerobic and anaerobic biodegradability potential; and OncoLogic that uses rule-based decision trees to evaluate the carcinogenic potential of chemicals. This model mimics expert judgment by following sets of knowledge rules based on studies of how chemicals cause cancer in animals and humans [12].
The next step is the development of systems that are able to predict tissue, organ, or whole animal toxicological endpoints, because one of the major issues in the use of in vitro assays to predict whole organism effects. The use of machine learning techniques that can help to uncover these often very complex relationships. For example, the “Merck Molecular Activity Challenge” in 2012 was won a group that used deep neural networks to predict the biomolecular target for specific chemical compounds [13], and the “Tox21 Data Challenge” in 2014 that was also won by a group the used deep neural network to detect off-target and toxic effects of environmental chemicals [14].
This demonstrates that machine learning techniques have the potential to model complex biological data to support drug discovery, toxicological research, and the development of new chemicals, with a return on investment in terms of reducing the costs of laboratory testing and the need for animal testing.
Making the change through seeing the return on investment
Machine learning is applicable to a broad range of challenges in many fields of chemistry, but making the change is about seeing the potential in terms of a company’s return on their investment. Machine learning techniques have the potential to draw returns in terms of improved time efficiency, increased prediction accuracy, and better cost effectiveness. Its application in chemical discovery and design can help identify more sustainable manufacturing techniques, e.g. improved environmental performance through reducing unwanted toxicity and making energy savings.
Embracing new technological advances to help deliver more sustainable and greener solutions is smart for business in the chemical industry. For example, the environmental effects of pesticides are now part of the customer consciousness and can influence customer purchase behaviour. We anticipate that machine learning algorithms will be a valuable tool for the chemical industry sector for years to come.
References
[1] Goh et al., Deep Learning for Computational Chemistry https://arxiv.org/ftp/arxiv/papers/1701/1701.04503.pdf
[2] Salahinejad M, et al. (2013). Capturing the crystal: prediction of enthalpy of sublimation, crystal lattice energy, and melting points of organic compounds. J Chem Inf Model. 53:223e9.
[3] Saldana DA, et al. (2012). Prediction of density and viscosity of biofuel compounds using machine learning methods. Energy Fuels. 26:2416e26.
[4] Chou, et al. (2014) Machine learning in concrete strength simulations: Multi-nation data analytics. Construction and building materials. 73. 771-780.
[5] Shandiz and Gauvin. (2016). Application of machine learning methods for the prediction of crystal system of cathode materials in lithium-ion batteries. Computational materials science. 117, 270-278.
[6] Khan, et al. Correlating dynamical mechanical properties with temperature and clay composition of polymer-clay nanocomposites.
[7] Lüti et al. (2016). The quantum chemical search for novel materials and the issue of data processing: The InfoMol project. Journal of computational science. 15. 65-73
[8] Rupp M (2015) Machine learning for quantum mechanics in a nutshell. Quantum Chemistry. 116 (16): 1058-1073 http://onlinelibrary.wiley.com/doi/10.1002/qua.24954/full
[9] Rupp, M. et al. (2012) Phys. Rev. Lett. 108.
[10] Montavon, G. et al. (2013). New J. Phys. 15.
Chemical Industry
[11] https://www.epa.gov/tsca-screening-tools/download-epi-suitetm-estimation-program-interface-v411
[13] Ma J, et al. (2015) Deep neural nets as a method for quantitative structure–activity relationships. J Chem Inf Model 55:263–274. http://citeseerx.ist.psu.edu/viewdoc/download?doi=10.1.1.704.5296&rep=rep1&type=pdf
[14] Andreas Mayr GK, et al. (2016). DeepTox: toxicity prediction using deep learning. Front Environ Sci 3:80.
Image references
ZackWeinberg https://commons.wikimedia.org/wiki/File:Svm_separating_hyperplanes_(SVG).svg
Zufzzi https://commons.wikimedia.org/wiki/File:Neural_network_bottleneck_achitecture.svg
Chemical Industry – Please read our blog post about
Smart Predictive Maintenance – The Key to Industry 4.0