Companies have always been very interested in expanding and improving their decision-making principles. In the past, business decisions were largely based on the experience of proven employees and gut instincts.
Why switch to Predictive Analytics
Over time, accounting systems have become easier and better at revising past business data for interesting patterns and deviations. The problem with these analyzes, however, was that they were always focused on the past. More than one “Lessons Learned” was not in it. Future developments were usually derived from more or less arbitrary conclusions.
This is precisely the difference between predictive analytics and traditional analysis techniques. Following the determination of patterns, future predictions are calculated using modern algorithms and models. Subjective assumptions have served their purpose or are being reduced to a minimum.
Predictive Analytics – Areas of Application
The emergence of predictive analytics is primarily due to the rapid growth of enterprise and Internet data volumes. It is therefore not surprising that the financial sector, which has been dealing with large amounts of data for a long time, introduced such procedures more than 20 years ago. Meanwhile, predictive analysis is used in many industries. Be it in marketing, healthcare, aerospace, etc. The aviation industry, for example, has been using such methods for quite some time to optimally align fares and available seats. The predictive analysis is also very often used in the field of customer loyalty and acquisition. Data from customer relationship management systems can be used to generate forecasting models that analyze customer and termination behavior and can thus be used to improve customer loyalty and customer acquisition. Another very exciting and seminal field of predictive analysis is Predictive Maintenance. With this section, we want to deal in more detail in one of the next blog articles.
The most important prerequisite for a successful predictive analysis is reliable data or high data quality. One can only calculate a reliable forecast if the quality of the database is high.
In the graphic below, we have compiled an overview of the most proven algorithms that are mainly used in the field of predictive analytics.
6 Predictive Analytics Algorithms
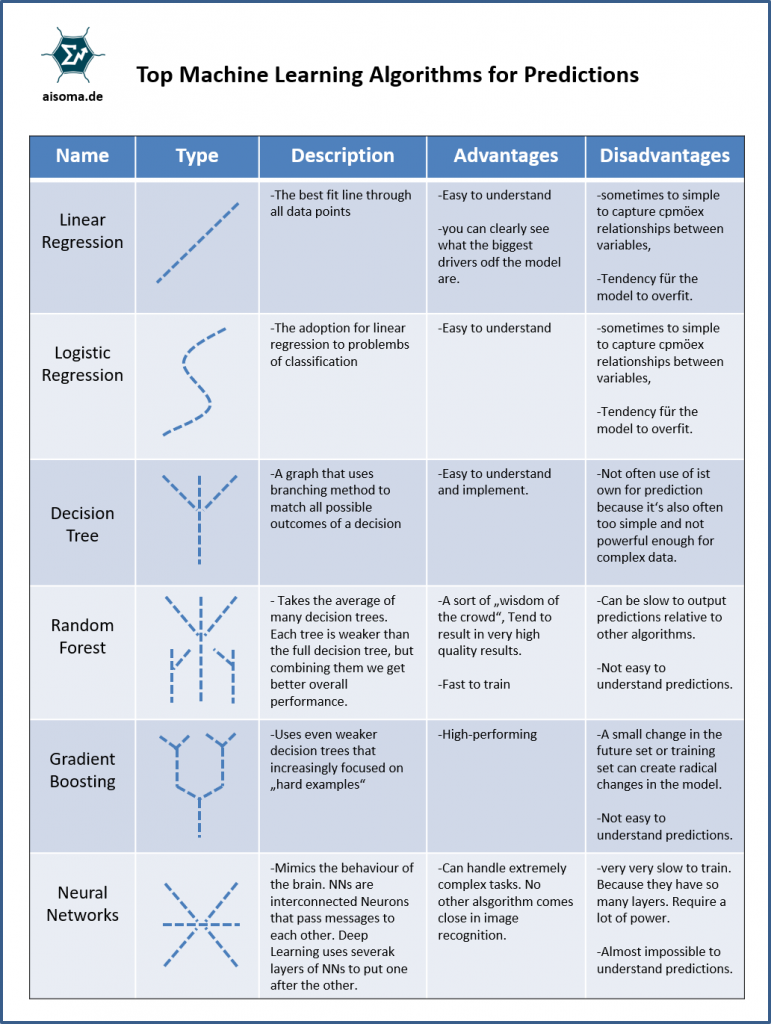
In order to calculate more meaningful and accurate predictions, it usally requires the combination of several algorithms and models. For this, the data scientist should already have a lot of experience. In addition, the data must be transformed into a suitable data structure before it can be used for predictive analysis. This process is one of the biggest challenges of predictive analysis and should be done by experts only.
AISOMA
Murat Durmus (Author of the Book: THE AI THOUGHT BOOK)
An excerpt of the book can be downloaded here: THE AI THOUGHT BOOK
Recommended Book: Taxonomy of the most commonly used Machine Learning Algorithms